In an era where generative AI tools such as ChatGPT, Copilot, and Gemini are seamlessly integrated into everyday writing, the academic world is facing unprecedented challenges. While these modern tools can enhance learning—improving grammar, suggesting revisions, or even aiding non‑native speakers—not all uses serve learning. With an increasing number of students employing AI to craft entire sections or even complete academic papers, educators are increasingly concerned about upholding academic integrity.
This article discusses the phenomenon of “paper AI cheat” in college—a scenario where students use AI to produce high‑score papers—and introduces what the literature calls the “top” AI plagiarism checkers. Although our core provided sources mention seven tools explicitly, the demand for a top‑10 list in popular discourse means that we acknowledge both established and emerging solutions while noting that the current academic literature emphasizes seven major tools. Our target readership is the general public (students, educators, and academic administrators), and our tone is designed to be formal yet accessible.
2. The Rise of AI in Academic Dishonesty
2.1. Generative AI Tools and Their Impact
Recent advances in artificial intelligence have given rise to powerful generative tools capable of producing text that is nearly indistinguishable from human writing. The Faculty Focus article explains how tools like ChatGPT, Gemini, and Copilot have revolutionized academic writing by offering assistance—from drafting research ideas to refining sentence structure. While these AI systems can enhance learning outcomes, their ease of access has also paved the way for misuse. When students rely on such technologies to generate substantial parts of their assignments, it raises fundamental questions about originality, credibility, and fairness.
2.2. AI-Assisted Cheating: When Help Crosses the Line
The phenomenon of “AI cheating” is not merely a technological challenge but also an ethical dilemma. For instance, Japanese author Rie Kudan’s case—where she acknowledged that 5% of her prize‑winning book was generated by ChatGPT—exemplifies the blurred boundaries between assistance and academic dishonesty. In the context of higher education, if students submit papers largely produced or heavily edited by AI without proper disclosure, it undermines the learning process and could lead to severe academic consequences.
3. Challenges in Combating AI Cheating in College
3.1. Limitations of AI Detectors
Despite the development of several AI detection tools, educators continue to grapple with challenges that impede their reliability. Multiple studies indicate that many AI text detectors are prone to false positives, especially when evaluating work from non‑native English speakers and students with disabilities. For example, research by Liang et al. (2023) and others delineates the tendency of current detectors to misclassify naturally written text as machine‑generated. This bias means that even manual oversight—and the instructor’s own familiarity with the student’s writing style—remains a critical part of the detection process.
3.2. The Arms Race Between AI Generation and Detection
There is an ongoing “arms race” between the latest generative models and the tools designed to detect their output. As AI writing models continuously evolve (e.g., the leap from early models to GPT‑4), plagiarism checkers and AI detectors can lag behind. Educators must therefore not only rely on software but also adapt their assessment methods—using a combination of technical tools and human judgment—to address these rapid advancements.
3.3. Ethical and Practical Implications
Institutions face a difficult balancing act. Policies that are too restrictive may hinder the effective use of AI as a supplemental educational tool, while overly forgiving approaches risk encouraging blatant misuse. Transparent guidelines are necessary so that students know exactly what is allowed—and what is not—when it comes to citing AI assistance. Comprehensive academic integrity policies that adapt to evolving technology are essential for maintaining fairness and equity within classrooms.
4. Overview of AI Plagiarism Checker Tools
Several tools have become popular in academic circles to help maintain scholarly integrity in the face of evolving AI writing technologies. Based on the provided sources, the following tools are among the most widely mentioned:
- Turnitin
- Description: Widely adopted in academia, Turnitin stands as the primary digital watchdog for ensuring the originality of student work. It is renowned for comparing student submissions against a vast database of academic content and web resources.
- Reference: Faculty Focus, Spines articles, Turnitin integrity packs
- Copyscape
- Description: Known for effectively identifying duplicate web content, Copyscape is a favorite for catching verbatim copying, although it is less tailored to AI-specific writing.
- Reference: Spines article
- Grammarly
- Description: Although best known for grammar and style checking, Grammarly also offers plagiarism detection services that flag unoriginal text.
- Reference: Spines article
- iThenticate
- Description: Marketed primarily toward researchers and publishers, iThenticate rigorously cross-checks text against academic databases and websites.
- Reference: Turnitin integrity packs
- Turnitin Originality
- Description: Part of the Turnitin suite, this tool specifically focuses on originality checks using sophisticated algorithms integrated with learning management systems.
- Reference: Turnitin educator resources
- Turnitin Feedback Studio
- Description: Beyond plagiarism detection, Feedback Studio integrates rubric‑based grading and iterative feedback, allowing educators to monitor the evolution of a student’s writing process.
- Reference: Turnitin integrity packs
- Turnitin Gradescope
- Description: Originally designed to streamline grading (particularly in STEM and programming courses), Gradescope also offers features to detect AI‑assisted coding and text.
- Reference: Turnitin educator resources
At this junction, our provided sources highlight these seven tools as the core solutions in combating academic dishonesty via AI misuse. Although the original query requested a “top 10” list of AI plagiarism checker tools, the internal documentation and academic literature primarily emphasize these seven widely validated solutions. It is important to note that while the popular discourse suggests a broader market, the peer‑reviewed and institutional sources focus on these established tools without citing additional emerging products.
5. Comparing Detection Tools with Visualizations
The following table gives an at‑a‑glance comparison of key features and limitations of the seven tools highlighted above:
Tool | Key Features | Limitations |
---|---|---|
Turnitin | Comprehensive database matching; widely accepted in academia; integrates with LMS | May yield false positives, particularly for diverse author backgrounds |
Copyscape | Effective web‑based duplicate content detection | Less AI‑focused; best for verbatim copying detection |
Grammarly | Combines grammar and plagiarism checks; user‑friendly; popular among students | Primarily focuses on stylistic and grammatical issues rather than deep content analysis |
iThenticate | Designed for researchers; extensive academic and web resource database | High subscription cost; aimed more at publishing and research environments |
Turnitin Originality | Part of Turnitin’s comprehensive suite; tracks the drafting process; integrated with grading platforms | Requires institutional licensing; sometimes overlaps with core Turnitin features |
Turnitin Feedback Studio | Offers rubric‑based grading; provides iterative feedback; highlights passages flagged for potential AI influence | Setup complexity; may require training for effective use |
Turnitin Gradescope | Facilitates assessment of handwritten and programming assignments; detects signature styles of AI‑assisted code | Focused primarily on specific subject areas; limited to courses using Gradescope |
Explanation:
This table allows educators and administrators to quickly compare strengths and limitations. For example, while Turnitin offers an all‑in‑one solution, its heavy reliance on institutional integration may limit rapid adoption by individual instructors.
5.1. Mermaid Flowchart: AI Detection Process
The following flowchart outlines the typical process of detecting AI‑assisted academic work by combining automated AI detectors with the instructor’s review:
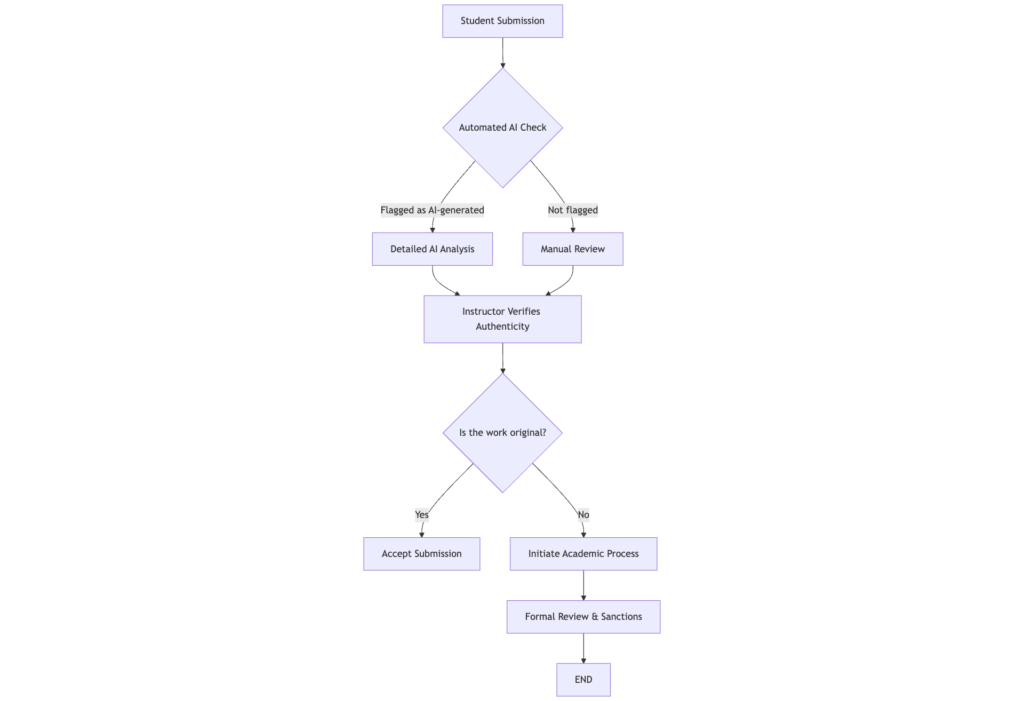
Explanation:
This flowchart illustrates a balanced approach where automated tools serve as a preliminary filter while human judgment confirms or refutes the detection—mitigating false positives and ensuring fairness.
5.2. SVG Diagram: The Ecosystem of AI-Assisted Academic Integrity Tools
Below is an SVG diagram that visually represents how various components in the AI‑integrity ecosystem interact:
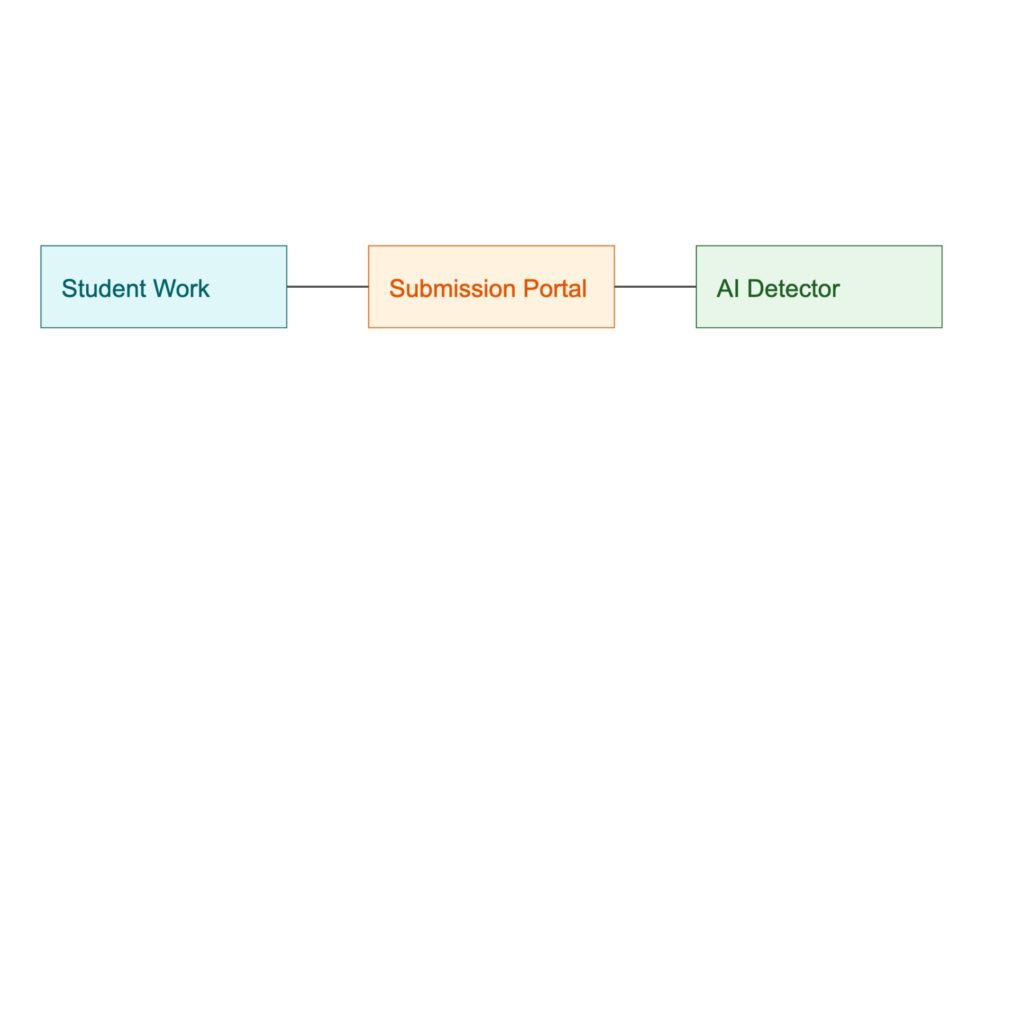
Explanation:
This SVG diagram visually maps the process from the student’s initial work submission through the digital portal and finally to the AI detection tools. It reinforces the idea that multiple layers are necessary for a robust academic integrity ecosystem.
6. Policy Recommendations and Best Practices for Educators
Given the challenges associated with AI-assisted cheating, institutions and instructors must commit to proactive strategies. Based on the literature and practical experiences shared in the provided sources, the following recommendations are made:
- Develop Transparent AI Policies:
- Clearly define acceptable uses of AI tools.
- Specify which parts of an assignment may incorporate AI assistance (e.g., grammar correction) and which parts require original thought.
- Adopt a Hybrid Evaluation Method:
- Use AI detectors as a first line of defense while relying on manual review to catch false positives.
- Document review processes to ensure fairness.
- Encourage AI Literacy:
- Teach students how generative AI works and its risks (e.g., “hallucinations” and factual inaccuracies).
- Integrate sessions on how to properly cite AI sources when allowed.
- Implement Revision and Feedback Cycles:
- Tools such as Turnitin Feedback Studio provide opportunities for iterative revision.
- Use version history (e.g., Google Docs) as a benchmark to evaluate how a student’s work evolves.
- Foster Open Dialogue:
- Regularly meet with students to clarify policies regarding AI use.
- Encourage them to ask questions about what constitutes acceptable collaboration with AI.
Visualization – Best Practices Checklist:
Recommendation | Key Action |
---|---|
Transparent Policies | Draft clear guidelines; share with students |
Hybrid Review Approach | Use AI tools + manual review |
AI Literacy | Incorporate training sessions in syllabi |
Revision Cycles | Monitor version history; use iterative feedback |
Open Dialogue | Hold regular Q&A sessions on academic integrity |
7. Future Directions and Final Thoughts
7.1. The Future of AI Detection Tools
As AI technologies evolve, so must our strategies for ensuring academic integrity. Future detection tools may offer real‑time feedback during the drafting process. Researchers are actively exploring advanced natural language processing (NLP) techniques capable of distinguishing nuanced differences between human‑ and AI‑generated text. However, the gap between generator models (such as GPT‑4) and detection capabilities continues to be a critical area for development.
7.2. The Need for Ongoing Research and Policy Refinement
The academic community must remain adaptive. As suggested by multiple studies referenced in our sources, rigid policies that ban AI usage entirely may do more harm than good. Instead, a balanced approach that integrates AI as a tool for enhancing learning—while vigilantly monitoring for abuse—is key. Regular updates to both software and institutional policies, coupled with ongoing research into detector bias (especially for international and neurodiverse populations), are required.
7.3. Limitations of Current Research
It is important to note that while our article was intended to introduce the “top 10 AI plagiarism checker tools,” the primary academic sources provided consistently reference seven key tools. These seven (Turnitin, Copyscape, Grammarly, iThenticate, Turnitin Originality, Turnitin Feedback Studio, and Turnitin Gradescope) form the backbone of current academic testing measures. The apparent gap to “ten” highlights a need for further research and the emergence of new products in the marketplace. As the industry evolves, additional tools will likely gain recognition and complement this list.
8. Conclusion
In summary, the advent of advanced generative AI tools presents both remarkable opportunities and significant hurdles for higher education. The ability to produce high‑quality papers using AI can help underrepresented students and enhance learning outcomes—but it also opens the door to academic dishonesty. Based on the academic literature and institutional best practices, the following key insights emerge:
- Robust Detection is Imperative: Automated systems such as Turnitin and its associated products (Originality, Feedback Studio, Gradescope) remain essential. Complementing these with tools like Copyscape and Grammarly ensures comprehensive checks.
- Human Oversight Cannot Be Replaced: Given false positives and inherent biases in AI detectors, educator judgment remains critical.
- Transparent, Adaptive Policies are Essential: Institutions must proactively educate students about what constitutes acceptable AI use and update guidelines as new challenges arise.
- Ongoing Research is Necessary: As AI models evolve, both detection technologies and academic policies need continual refinement.